The starter pistol for AI has fired and cooled.
The smoke has cleared, and organisations everywhere are starting to dig in for the long-term and take their corners carefully. If anything, this is the more exciting period: the noisy furore has ended, and we are now seeing the real power and value that AI can deliver.
However, it’s important to keep in mind that the actions we take today also shape the industry’s future direction and governance. As such, we should bear in mind the ‘Spiderman factor’- that with great power comes great responsibility – and make sure that we’re creating, hosting and maintaining AI responsibly. This isn’t just a nice to have: being responsible with AI not only reinforces customer trust, but also avoids potential legal and regulatory issues further down the road.
What is Responsible AI?
There are a number of definitions, but at the end of the day, responsible AI is what it says on the tin. AI needs to be created and maintained with the utmost respect for ethical and legal processes. According to organisations like the World Economic Forum, the Alan Turing Institute and the National Institute of Standards and Technology, this includes both technological and social factors, for example:
– Bias and Fairness: AI systems should use data that has been fairly selected and considered to avoid bias. In many cases, society has deeply entrenched and intrinsic biases, and testing groups must be assembled from a diverse range of backgrounds to help avoid replicating this in AI systems.
– Transparency and Accountability: AI is often described as a black box, but where possible, systems should be explainable and transparent. Similarly, the data that models have been trained on should be ethically obtained and free from copyright or intellectual property issues.
– Robustness and Security: AI systems should perform reliably – they should not give two different answers to the same query, for example. They should also be secure from outside interference.
– Privacy: AI should protect personal data, and it should be clear how AI feedback mechanisms work – for example, it should be made clear if user input data will later be used to further train and refine the model.
– Avoidance of Harm: AI systems should not be used to harm individuals directly or indirectly. Acts like the EU AI act have explicitly highlighted certain areas where AI is either forbidden (for example AI that uses subliminal systems or adversely affects someone’s ability to make a reasonable and balanced decision) or where extreme care and supervision should be taken when developing it.
With these factors in mind, it might seem that keeping AI models within the four walls is the best thing to do from a control perspective. Basic data sovereignty practices for both non-AI and AI systems tell us that it’s important to consider where data is hosted, for a number of reasons. For example, many countries have practices where data hosted in local datacentres can be inspected for political or economic intelligence purposes.
However, cloud environments do offer a number of benefits. As we all know, the processors needed to train AI models are enormously expensive, and being able to rent servers on a per-minute or hourly basis is far more cost-effective than purchasing one outright. This agility is incredibly useful to AI organisations, many of whom are startups with limited access to up-front capital.
At the same time, having access to top-spec GPUs on a pay-as-you-go basis also means that tests can run faster. Organisations can train their models, evaluate the outputs and evolve the model far faster on a high-performance GPU than on the lower-spec model that they might have been able to buy outright. In the startup world – especially in the AI startup world – speed is crucial, and delivering a high-quality MVP can be an essential step towards revenue, not to mention investor confidence. And with due care and attention, AI can live comfortably and responsibly in the cloud.
AI in the Cloud
As we’ve said, there are both benefits to be realised and pitfalls to be avoided when migrating AI to the cloud. Cloud providers offer high-spec, affordable infrastructure, often with better security arrangements than on-premises systems can provide – not to mention the capability to handle routine patching and updates. But there are a number of other factors to be mindful of, including:
– Sovereignty: In many cases, it doesn’t matter where models are being trained, data transfer fees permitting. Compute in one area may be significantly cheaper than in another, but if you’re moving data to another country it’s important to understand how data will be handled there, including any differences in governmental or security process.
– Sustainability: It’s also important to know how sustainable and power-efficient your AI cloud partner is, particularly if you’re transferring data to another country. Some countries have very good renewable energy mixes – but others don’t, and some datacentres are intrinsically more efficient than others. Do remember that your AI cloud provider will form part of your scope 3 emissions, so it pays to do your due diligence, particularly since AI can be very power hungry.
– Suitability: The kind of data that your AI system is processing will have an impact on the kind of environment that it needs. For example, are you handling payments or patient data? Some cloud providers can offer infrastructure environments and processes that are tailor-made for these kinds of application – but others can’t.
– Openness: Many organisations will have a lot of their technology stack in one cloud, but host AI in another because of GPU pricing or availability. If you’re going to be moving data from one place to another, it may make most sense to work in an open-source environment for ease of migration. However, it’s also important to be aware of any ingress or egress fees when you do – and even though many of the cloud providers have reduced or removed their fees, it’s always worth checking the small print.
Today we’re really starting to see AI’s rubber hit the road across a number of industries, including both generative and non-generative applications. However, organisations do need to make sure that they’re doing their due diligence both in and out of the cloud or they may risk getting caught in their own exhaust fumes.
About the Author
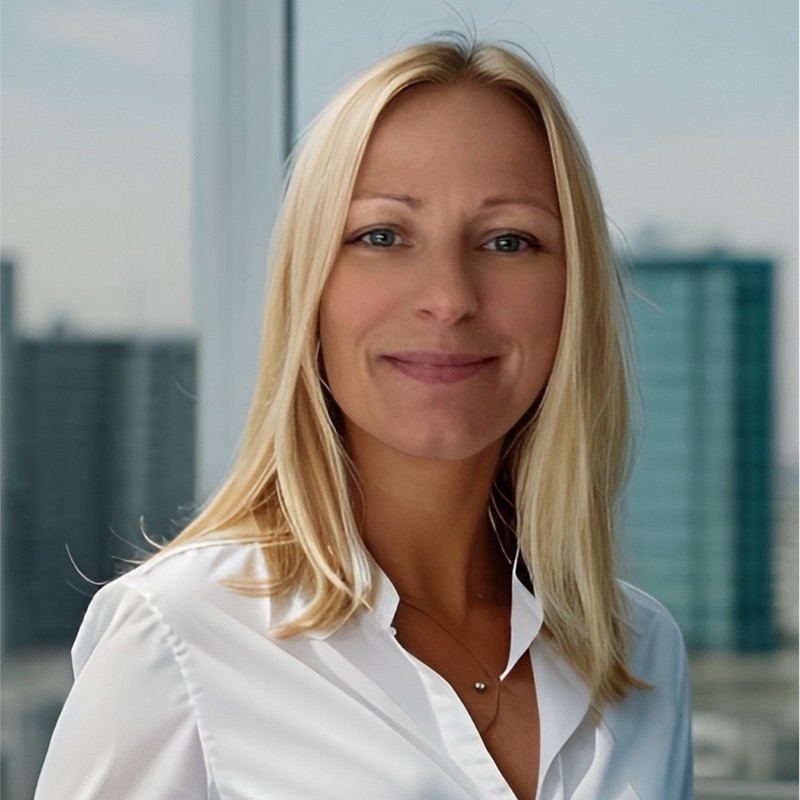
Emma Dennard is VP Northern Europe at OVHcloud. OVHcloud is a global player and the leading European cloud provider operating over 450,000 servers within 43 data centers across 4 continents to reach 1,6 million customers in over 140 countries. Spearheading a trusted cloud and pioneering a sustainable cloud with the best price-performance ratio, the Group has been leveraging for over 20 years an integrated model that guarantees total control of its value chain: from the design of its servers to the construction and management of its data centers, including the orchestration of its fiber-optic network.
Featured image: Adobe Stock