Industry-specific GenAI, the role of a unified data strategy and the importance of responsible and secure AI are three trends that will define the GenAI market
I have no doubt that the world has entered the artificial intelligence era, with its subset generative AI (GenAI) making waves across almost every industry. How to get the most out of GenAI in a safe, secure and scalable manner dominates almost every conversation I have with our enterprise clients.
According to the 2024 Gartner CEO survey, AI has now emerged as the differentiating technology for delivering corporate strategies, including revenue generation and operations, with 24% prioritizing the tech compared to just 4% in 2023.
The rise of GenAI
Since GenAI exploded onto the scene at the end of 2022, with OpenAI’s release of ChatGPT, executive leaders — many of whom had not heard of the technology before — have been quick to adopt the tech and increase spend in this area.
According to Gartner’s GenAI in Technology Services research, the five-year CAGR for AI is forecast to grow by 17% between 2023-2027 and much of this will displace spending in other IT categories.
Organizations are looking to replace current IT strategies and plans with “AI First” strategies, while they are planning to deploy the technology in the same way as previous innovations, with a mixture of buying and building GenAI models, services and solutions.
With the emergence of any new technology that is creating such a significant impact, it’s important to understand the trends shaping the market. In this article, I will break down three key trends propelling GenAI to the forefront of creating competitive business advantage.
- Industry and domain-specific GenAI
Just as we’ve seen with the rise of industry cloud, GenAI models will increasingly be built for specific industries and business functions.
According to Gartner, this will apply to more than 50% of GenAI models by 2027 — up from approximately 1% in 2023. This trend is driven by the need for more precise and contextually aware AI applications in various industries.
Different industries have distinct needs and like with cloud, standardized or general GenAI models and services can’t support the specialized requirements of specific industries. This is especially true for regulated industries that have stringent governance, risk and compliance standards — industry or domain-specific GenAI models will help organizations comply with regulations and compliance standards, ensuring data security and ethical considerations are adhered to.
GenAI tailored to specific industries or domains learn from smaller datasets or small language models (SLMs) that often require less computational power than training general-purpose models, like OpenAI’s ChatGPT or Google’s Gemini. This approach enables them to understand industry of domain-specific language or subtleties better, which will lead to better outputs.
For example, in healthcare, Google Research has developed a large language model (LLM) called Med-PaLM. This LLM is designed to provide high quality answers to medical questions. The second version, Med-PaLM 2, is one of the research models powering MedLM, which is a family of foundational models created for the healthcare industry. Med-PaLM 2 achieved an accuracy of 85.4% in US Medical Licensing Exam-style (USMLE) questions, surpassing GPT-42. It is the first language model to achieve expert-level performance on USMLE-style questions with more than 85% accuracy.
In the retail industry, RetailGPT is creating a new era of phygital shopping and dining through the power of GenAI. Through an app, RetailGPT provides an incredibly personalized and conversational experience for customers, while enabling retailers to better understand and engage their customers through data.
These emerging use cases extend to almost every industry and the strategic deployment of GenAI can not only enhance existing offerings but pave the way for new products, services, revenue streams and future innovations.
- A unified data strategy
To train any AI model data is needed and it is a foundational element.
High-quality and large volumes of data are fundamental in generating impactful outputs that can be used to create meaningful products and services. As a result, today, organizations are prioritizing acquiring and managing data.
This, however, is a significant challenge for many reasons, including siloed data, AI and infrastructure teams, inconsistent data quality, increasingly stringent data privacy laws that adds new levels of complexity, a lack of large, diverse datasets to train the models and the presence of bias in datasets, which are then baked into AI models. This leads to biased outcomes and potentially unfair decisions, such as being turned down for a mortgage due to the color of your skin.
These types of challenges mean that organizations might incur higher costs and potential model limitations when training AI models.
To overcome these issues, organizations should focus on creating a unified data strategy linked to AI, infrastructure and crucially, business outcomes. This should include a hybrid approach to data acquisition and management, combining synthetic and real-world data, while practicing continuous evaluation with human oversight to address the challenges of data quality, variety, stringent regulation and bias.
- Responsible and secure AI
With the accelerated adoption of GenAI, responsible and secure AI has become paramount.
Responsible AI is the practice of ensuring AI systems are created and deployed in an ethical way.
The main reason for prioritizing responsible AI is to mitigate bias. Mitigating bias is fundamental in delivering GenAI solutions that have true market applicability and relevance.
Ultimately, bias comes from three areas; algorithms, data and humans. Bias from AI algorithms has plummeted exponentially in the last decade. Today, algorithms are mostly trustworthy and the biggest source of bias in AI comes from data and humans.
When it comes to data, bias exists because of a lack of quality and variety, as well as often incomplete datasets used to train the algorithm. With humans, there is an inherent lack of trust when it comes to AI, whether because of reported threats to people’s livelihoods or due to AI hallucinating certain information.
While responsible AI focuses on developing an ethical and unbiased AI environment, secure AI refers to measures that protect AI infrastructure and the data that supports these systems from cyberattacks.
Processing more data to train the algorithms creates a larger attack surface area and increases the likelihood of data breaches and leaks. Bad actors are also utilizing GenAI to create harder-to-defend, more intelligent cyberattacks.
Overcoming these various hurdles and implementing responsible and secure AI requires organizations to develop a framework that prioritizes areas like explainability, fairness, transparency, privacy and security.
Many IT service providers have created frameworks to help enterprises adopt responsible and secure AI practices. HCLTech is no different. Our AI Force platform aims to help organizations embrace responsible AI by integrating robust security and governance measures to foster safe and secure innovation at scale.
Defining the GenAI market
While the GenAI market is in its infancy, the implications for the future of business and society are significant.
GenAI and AI are now strategic priorities for organizations, with Gartner’s 2024 CEO survey finding that 59% of CEOs agree that AI is the technology that will most impact their industry.
Will the technology behave like other emerging technologies; hype followed by the trough of disillusionment? That remains to be seen.
What is clear is that to survive and thrive, organizations will need to identify tangible business use cases, upskill and reskill employees, focus on disciplines like prompt engineering, and shift organizational structures to accommodate a new world, where GenAI augments human work, ingenuity and decision-making.
To do this, forward-looking enterprises will need a GenAI partner that understands their unique business requirements and has a track record of delivering safe, scalable and high-performing AI solutions, as well as a comprehensive suite of GenAI-powered services.
About the Author
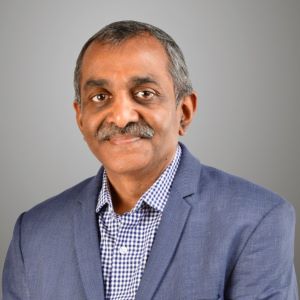
Vijay Guntur is Chief Technology Officer at HCLTech. HCLTech is a global technology company, home to more than 219,000 people across 60 countries, delivering industry-leading capabilities centered around digital, engineering, cloud and AI, powered by a broad portfolio of technology services and products. We work with clients across all major verticals, providing industry solutions for Financial Services, Manufacturing, Life Sciences and Healthcare, Technology and Services, Telecom and Media, Retail and CPG, and Public Services.
Featured image: Adobe